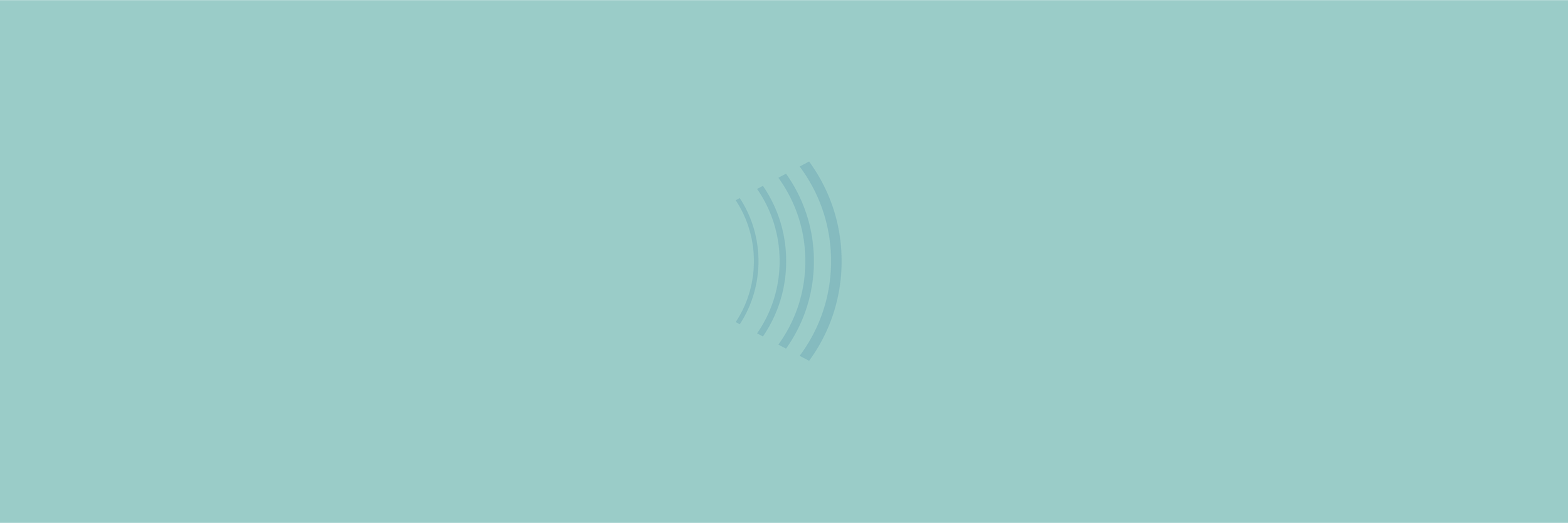
Background
HeardThat is a smartphone app that is designed to help consumers better understand conversations in the presence of noise. The app uses AI on the phone to remove ambient noise and deliver clean speech to the user’s ears via earbuds, headphones, hearing aids, or cochlear implants.
This is a preliminary report as part of an ongoing study with listeners to measure the efficacy of the HeardThat app for improving understanding of speech in noise while reducing listening effort. The participants are listeners who report having difficulty hearing in background noise.
Does the app work?
When a new product like HeardThat is released to the market, it is natural to ask for evidence that it works. There are different ways to address this. Efficacy refers to how well something works in a controlled setting. This is in contrast to effectiveness, which is how well it works in the “real world”. Ultimately, the consumer or professional wants to know the effectiveness of the product, but there is value in isolating its efficacy.
Hearing is the result of complex physical and mental processes, many of which are not well understood. It can be challenging to measure intelligibility and listening effort, as experienced by the listener, in an objective way.
As the developers of HeardThat, we have used a variety of approaches to assess whether the app is effective:
mathematical measures of audio fidelity that are used as part of the AI training process
independent measurements using the industry-standard 3QUEST and ABLE algorithms
our own experience of using the app
in-depth collaboration with a small number of users
informal tests with focus group participants
hundreds of in-person demos
feedback from publicly available free trial
To answer a prospective user’s question, “Does the app work?”, we have taken the approach of making it as easy as possible to try the product. It is easily obtained from the app stores and it requires no new devices because sound is delivered to the user’s ears through their earbuds, headphones, hearing aids, or cochlear implants. The app just takes a couple of minutes to download, install, and try.
But even before deciding to try the app, prospective users (and others) can
explore an interactive demo
listen to recorded demos
The intention is to be as transparent as possible about what the user should expect.
Efficacy study
Goals
To supplement the “try it” approach, we also wanted to provide statistical evidence based on user studies. To this end, we devised an efficacy study that pays particular attention to the user’s experience of hearing when using the app, for real-world sounds.
In other words, we wanted to devise an ecologically valid speech-in-noise test, one that is representative of real-world auditory ability. Moreover, the test was conducted with the user's own phone and preferred listening devices (as would be the case with the HeardThat app).
The goal is not to assess the subject’s hearing but rather to assess the ability of the app to achieve its goals.
Experimental design
The study is based on participants listening to Harvard sentences and repeating back what they heard. There were 20 test sentences recorded by a male speaker and 20 different test sentences recorded by a female speaker.
Clean: The sentences were recorded in a quiet room
Noisy: The sentences were recorded in a cafe with ambient noise levels of approximately 75 - 80 dB SPL. The recordings for this set were made on a microphone cluster located at the position of the HeardThat user.
At the same time as Set #2 was recorded, recordings were also made on a phone running HeardThat. The app was used in the usual way: the phone was laid on the table at a location in front of the HeardThat user with the HeardThat app open on the screen and the Start button activated. The phone was about 61 cm (24”) in front of the talker and 41 cm (16”) down.
The output from HeardThat made up the final set of test sentences.
Processed: The sentences spoken in the noisy cafe, after processing by HeardThat.
The sentences used in the test were equally divided between the male and female speakers. Different sentences were used for each speaker and each set, for a total of 30 sentences overall.
To conduct the study, the users downloaded an app. The app played the recorded sentences and recorded the user’s verbal repetition of what they heard. The listeners used their choice of earbuds and headphones and set the volume to a level that was comfortable for them. The 10 clean sentences were presented first to establish a baseline, then the 10 noisy sentences, and finally the 10 processed sentences.
Because the participants only had access to auditory cues, visual cues (speech reading, body language) were not a confounding variable. Another possible confounding variable is that participants could interpolate missed words based on semantic context. This effect is minimized by using the Harvard sentences because these sentences are designed to stand alone as single sentences that are semantically unusual. In other words, it is hard to infer their meaning if the listener missed some of the words.
At the end of each of the three test conditions, the participant was asked to rate the listening effort on a scale from 1 (least effort) to 10 (most effort).
Participant selection
Participants from among the company’s users and other contacts were invited to participate. Those who were selected reported they are able to hear adequately in quiet environments, but have difficulty understanding speech in background noise. They do not normally wear hearing aids or cochlear implants. (A future phase of the study will include this sub-population of participants.)
Based on previous interactions with HeardThat users, we expected the effect size to be large. Initial power analyses suggested that 10 participants should be sufficient to find a meaningful effect (over 90% power). In the end, 12 participants completed the testing protocol. All submissions received were included in the study.
The participants were adults aged 20 - 64 who reported being able to hear well in quiet locations, but have difficulty hearing in background noise. There were 5 female and 7 male participants.
Analysis
The recorded sentences repeated by the 12 participants for the three sets of test sentences were transcribed manually and compared with the original spoken sentences. Two measures of understanding were assessed and calculated.
First, the word error rate (WER) was calculated. This is an industry standard based on the number of deletions, insertions, and substitutions of words. It gives a measure of how well words were identified. It ranges from 0% - 100%.
However, WER has a number of well-known limitations because of its emphasis on individual words. Sentence identification is arguably a better indicator of ease of conversation.
To measure sentence identification in an objective way, we used text embeddings, a technique that is common in NLP (natural language processing) research. Embeddings convert text strings into vectors in a high-dimensional space, where semantically similar content is positioned closer together. We computed the embeddings for the original and repeated sentences, and then calculated the distance (CD = cosine distance) between them in the embed space. The CD is a floating point number between 0 (identical) and 1 (orthogonal). For ease of comparison, we converted this to a percent and normalized the result to be on a scale comparable to WER.
To understand why WER can be misleading, and how CD can be helpful for assessing speech understanding, consider this sample sentence that is repeated back in two different ways:
In case #1, the repeated sentence means something very different from the original but WER is low, which misses the problem. CD is relatively high indicating that the meaning has changed.
In case #2, the repeated response essentially has the same meaning as the original, but the WER score is quite high. The CD score is able to measure that the original and repeated sentences are similar in meaning and results in a better lower score.
We used WER and CD to measure the reduction in identification errors for words and sentences respectively, after adjusting for baseline performance on clean speech.
Results
Reduction in word understanding errors
Reduction in sentence understanding errors
Significant reduction in listening effort
Word and sentence errors
HeardThat reduces errors in identifying words by 76% and sentences by 85%.
Listening effort
All participants reported a reduction in listening effort between the noisy and processed speech. 82% of participants reported a significant reduction, as defined by 2 points in the 10-point scale. The average increase in listening effort between clean and noisy was 4.3 on that scale, whereas the average difference between clean and processed was only 0.5.
Significance
Findings with 12 participants were statistically robust (p < .001) with a clinically meaningful effect. This means that there is less than a 0.1% possibility that these findings could have been due to chance. The reason for such strong statistical significance is likely due to the fact that every single participant made fewer errors and reported lower listening effort for speech processed by HeardThat compared to speech in a noisy environment.
There was a large overall decrease in participants' error rates in speech processed by HeardThat compared to speech with background noise (word: t = -3.77, p < .001; sentence: t = -3.96, p < .001) and the effect sizes were very large (word: Cohen's d = 1.54; sentence: Cohen’s d = 1.62). The decrease in reported listening effort was similarly large (t = -4.80, p < .001) with a very large effect size (Cohen's d = 1.96).
Conclusions
The use of HeardThat to reduce the deleterious impact of background noise resulted in a very significant improvement in word and sentence comprehension. In addition, listening effort was essentially restored to a level close to that of a quiet room despite the fact that the sentences were recorded in a noisy coffee shop.
Our study's findings are consistent with the Framework for Understanding Effortful Listening (FUEL), which posits that hearing impairment increases the cognitive load required for speech comprehension. Enhancing the auditory input can alleviate the burden associated with effortful listening.
Findings from this efficacy study suggest that for a person with typical quiet-room hearing who experiences difficulty hearing speech in noisy environments, HeardThat does improve understanding conversations more easily in the presence of noise. Even more encouraging, HeardThat may help to understand conversations as well as if there was no noise at all.